Welcome to PDEP!
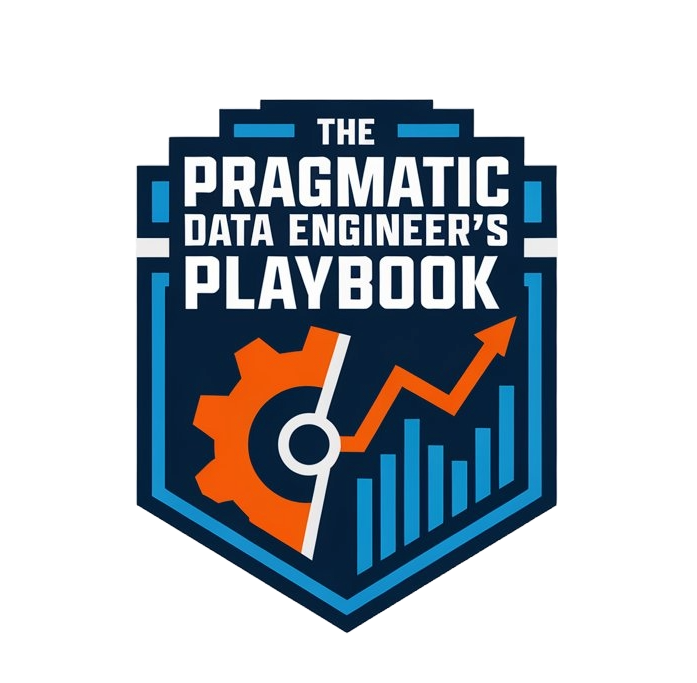
The Pragmatic Data Engineer's Playbook (PDEP) is a newsletter that deep-dives into complex Data Engineering Technologies, Distributed Data Systems, and Data Architecture. Its goal is to help you make
- better design decisions by picking the right technology for the job,
- help you grow technically strong to move to the next level in your career
- help you develop a deep intuition of how these technologies work internally. So, you can use this intuition to implement advanced solutions.
Who Is PDEP For?
- Data Engineers: You want to upgrade to the next level to get that job promotion and develop a deep intuition of how Data Engineering Tech works under the hood.
- Data Architects: You want to make good decisions while choosing the right tech for the job while building Data Architectures.
- Leaders or Directors in the Data Domain: You want to stay up-to-date with techs in the rapidly evolving Data Engineering Domain.
- For the Curious Engineers: You love to dive deep into the internals of Data Engineering or Distributed Systems Tech to quench that curiosity.
Who Am I?
Akashdeep Gupta writes the Pragmatic Data Engineer Playbook Newsletter.
I am a Professional Principal Data Engineer:
- With over a decade of experience in the Data Engineering and Distributed Systems Domain.
- Worked with over 15 clients and helped them build scalable, robust, cost-effective, optimized solutions on the cloud.
I firmly believe that now is the right time to develop a deep intuition, understand and leverage the internals of these technologies, and distinguish yourself in the rapidly evolving field of Data Engineering.
Best Issues!
Still not sure if PDEP is for you?
Here are some of the most-read issues so you can decide for yourself:
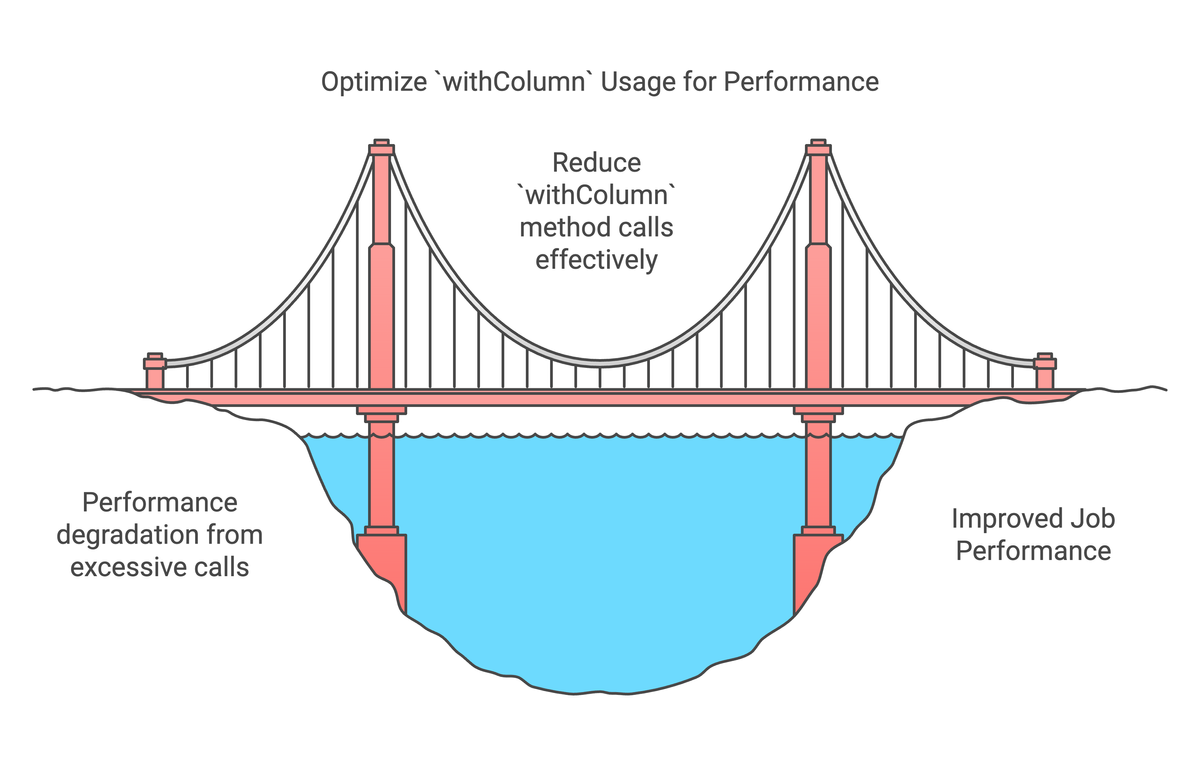
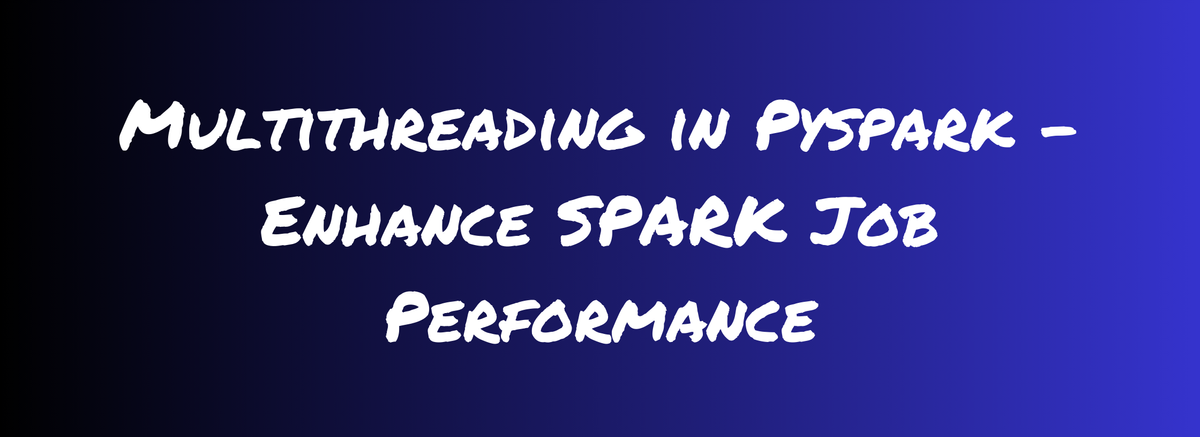
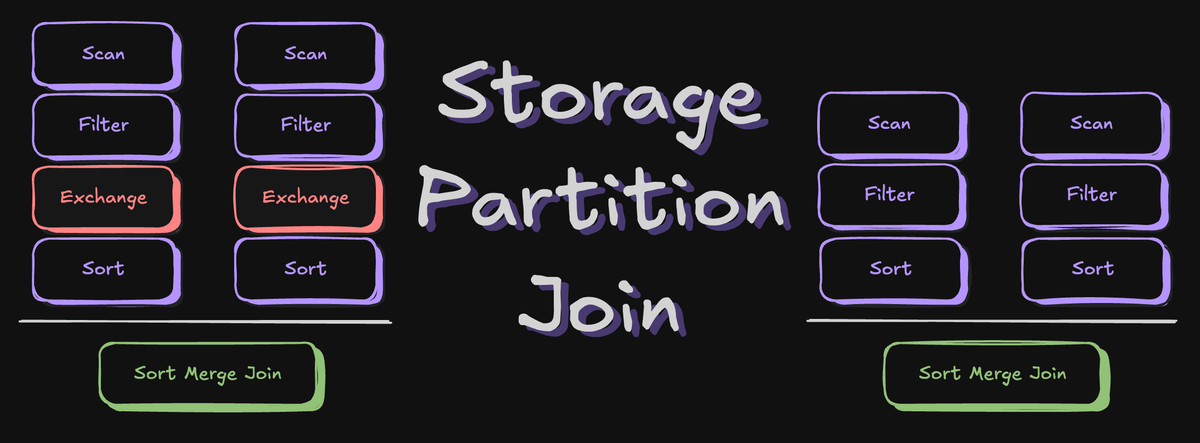
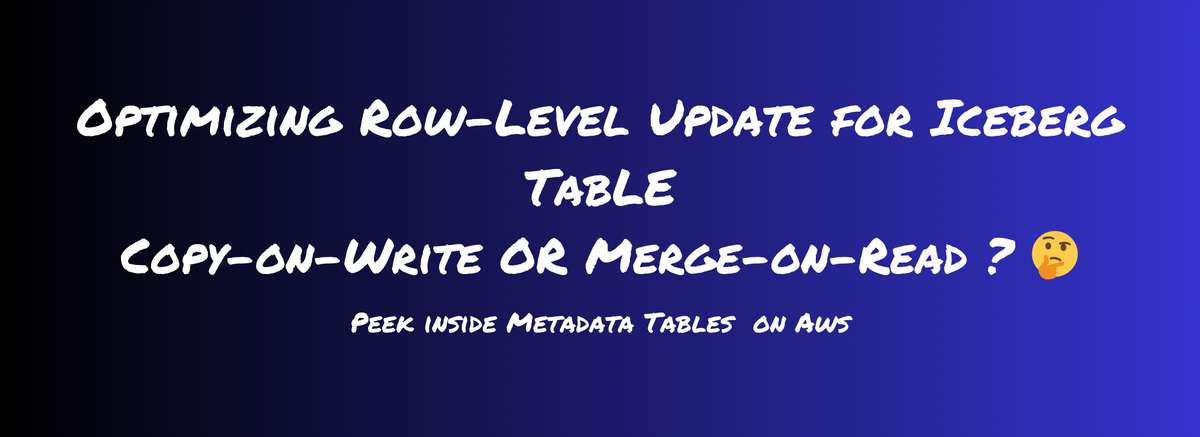
My Promise To You
As a PDEP reader, I promise:
- I will never miss 2 weeks in a row: Researching these techs takes serious time. I commit to delivering a newsletter every two weeks that provides meaningful, in-depth insights into data engineering technologies. No filler, no fluff.
- I will make sure each newsletter is worth your time: Every issue is carefully researched and crafted. I understand that your time is precious, so each newsletter is designed to provide real value that will make you move forward in your professional journey.
Each newsletter is something I'd want to read if I were in your shoes - practical, direct, and something I can actually use in my work. - I will re-iterate in real-time based on your feedback: Got a burning question? A complex problem you're stuck on? Shoot it my way. I'm not just throwing content into the void - I'm here to help you level up your data engineering game. Your feedback isn't just welcome, it's how I'll make this newsletter genuinely useful.
As a reader, you can check for new PDEP issues here on website—or, sit back and wait for them to hit your inbox as soon as it's published.